Overcome LLM Hallucinations Using Knowledge Bases
Hallucinations in LLMs can be avoided or reduced by enhancing the response using knowledge bases. Knowledge bases can be any of the organization's data. The LLM's response is grounded and a enhanced response is served to the user.
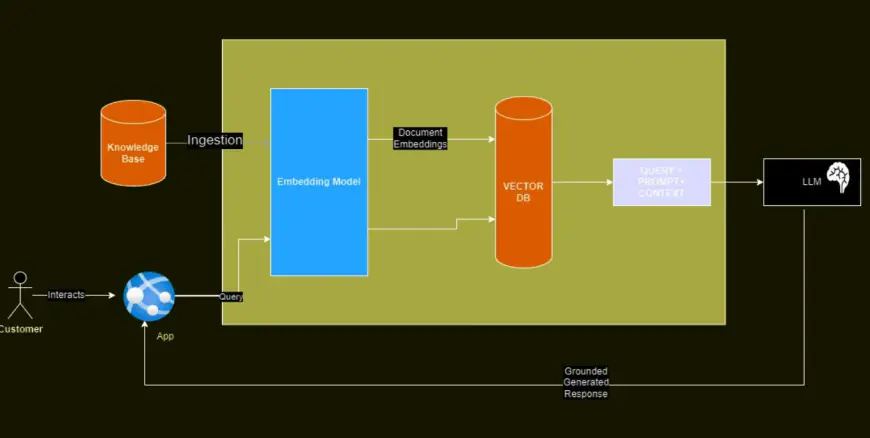
What?
If you prompt an LLM “suggest a great programming language for machine learning”
\ LLMs response would be: “One of the most recommended programming languages for machine learning is Python. Python is a high-level…”
\ What if you want your organization to provide a verified organization specific information i.e. enhance the response with authentic organization information?
\ Let us make it happen when interacting with LLM
Why?
Popular LLMs like OpenAI’s chatGPT, Google’s Gemini are trained on publicly available data. They often lack organization specific information. There are certain times where organizations would like to rely on LLMs. However, would like to enhance the response specific to a particular organization or add disclaimers when no grounding data is available.
\ The process of doing this is known as Grounding of LLM’s response using Knowledge bases.
How?
While, I can just talk about it.
\ As an engineer looking at some code snippets gives me confidence.
\ Executing them elevates my confidence and also gives happiness. Sharing gives me satisfaction :smile:
Code? Why not! → Python? Of course!!
- Install required libraries
pip install openai faiss-cpu numpy python-dotenv
openai
: To interact with OpenAI’s GPT models and embeddings.faiss-cpu
: A library by Facebook AI for efficient similarity search, used to store and search embeddings.numpy
: For numerical operations, including handling embeddings as vectors.python-dotenv
: To load environment variables (e.g., API keys) from a.env
file securely.
\
- Set Up Environment variables
- Navigate to https://platform.openai.com/settings/organization/api-keys
- Click on “Create new secret key” as show in the image below.
- Provide details, You can use a Service Account. Provide a name for the “Service account ID” and select a project.
- Copy the secret key to clipboard
- Create a
.env
file in your project directory. Add your OpenAI API key to this file.
OPENAI_API_KEY=your_openai_api_key_here
This file keeps your API key secure and separated from the code.
\
- Initialize client and load environment variables
load_dotenv()
loads the.env
file, andos.getenv("OPENAI_API_KEY")
retrieves the API key. This setup keeps your API key secure.
import os
from openai import OpenAI
from dotenv import load_dotenv
import faiss
import numpy as np
# Load environment variables
load_dotenv()
client = OpenAI(api_key=os.getenv("OPENAI_API_KEY"))
\
- Define Grounding Data/Knowledge base
- This dictionary contains the grounding information for topics. In reality, this could be a larger dataset or a database.
# Grounding data
grounding_data = {
"Python": "Python is dynamically typed, which can be a double-edged sword. While it makes coding faster and more flexible, it can lead to runtime errors that might have been caught at compile-time in statically-typed languages.",
"LLMs": "Large Language Models (LLMs) are neural networks trained on large text datasets.",
"Data Science": "Data Science involves using algorithms, data analysis, and machine learning to understand and interpret data.",
"Java": "Java is great, it powers most of the machine learning code, and has a rich set of libraries available."
}
\
- Generate Text Embeddings
- A function to generate embeddings for a given text using OpenAI’s embedding model. This function calls the OpenAI API to get the embedding for a text input, which is then returned as a NumPy array
# Function to generate embedding for a text
def get_embedding(text):
response = client.embeddings.create(
model="text-embedding-ada-002",
input=text
)
return np.array(response.data[0].embedding)
\
- FAISS Index and embeddings for Grounding Data
- Create a FAISS index, a structure optimized for fast similarity searches, and populate it with embeddings of the grounding data.
# Create FAISS index and populate it with grounding data embeddings
dimension = len(get_embedding("test")) # Dimension of embeddings
index = faiss.IndexFlatL2(dimension) # L2 distance index for similarity search
grounding_embeddings = []
grounding_keys = list(grounding_data.keys())
for key, text in grounding_data.items():
embedding = get_embedding(text)
grounding_embeddings.append(embedding)
index.add(np.array([embedding]).astype("float32"))
- \
dimension
: The size of each embedding, needed to initialize the FAISS index.index = faiss.IndexFlatL2(dimension)
: Creates a FAISS index that uses Euclidean distance (L2) for similarity.For each entry in
grounding_data
, this code generates an embedding and adds it to the FAISS index.\
- Vector search function
- Function searches the FAISS index for the most similar grounding data entry to a query.
# Function to perform vector search on FAISS
def vector_search(query_text, threshold=0.8):
query_embedding = get_embedding(query_text).astype("float32").reshape(1, -1)
D, I = index.search(query_embedding, 1) # Search for the closest vector
if I[0][0] != -1 and D[0][0] <= threshold:
return grounding_data[grounding_keys[I[0][0]]]
else:
return None # No similar grounding information available
Query Embedding
: Converts the query text into an embedding vector.FAISS Search
: Searches the index for the closest vector to the query.Threshold Check
: If the closest vector’s distance (D) is below the threshold, it returns the grounding information. Otherwise, it indicates no reliable grounding was found.
Query the LLM
We query the LLM using the OpenAI’s chatgpt api and gpt-4 model.
# Query the LLM
def query_llm(prompt):
response = client.chat.completions.create(
model="gpt-4",
messages=[
{"role": "system", "content": "You are a helpful assistant."},
{"role": "user", "content": prompt}
]
)
return response.choices[0].message.content
\
Enhanced response
- Appends grounding information if it’s available, or
- Adds a disclaimer if no relevant grounding information is found.
def enhance_response(topic, llm_response): grounding_info = vector_search(llm_response)
if grounding_info: # Check if the LLM's response aligns well with grounding information return f"{llm_response}\n\n(Verified Information: {grounding_info})" else: # Add a disclaimer when no grounding data is available return f"{llm_response}\n\n(Disclaimer: This information could not be verified against known data and may contain inaccuracies.)"
Define the main function
The main function combines everything, allowing you to input a topic, query the LLM, and check if the response aligns with the grounding data.
# Main function to execute the grounding check def main(): topic = input("Enter a topic: ") llm_response = query_llm(f"What can you tell me about {topic}?") grounding_info = vector_search(llm_response, threshold=0.8)
if __name__ == "__main__": main()print(f"LLM Response: {llm_response}") print(f"Grounding Information: {grounding_info}") if grounding_info != "No grounding information available": print("Response is grounded and reliable.") else: print("Potential hallucination detected. Using grounded information instead.") print(f"Grounded Answer: {grounding_info}")
Outcome
Execute the script
Invoke this snippet using
python groundin_llm.py
\ The response:
Explanation
If you notice the response, although the response from LLM was “One of the most recommended programming languages for machine learning…”, the grounded response was “Java is great, it power most of the Machine learning code, it has a rich set of libraries available”.
\ This is possible using Meta’s FAISS library for vector search based on similarity.
Process:
- First retrieve the LLMs response.
- Check if a our knowledge base has any relevant information using vector search.
- If exists return the response from "the knowledge base”
- If not return the LLM response as is.
\ Here is the code: https://github.com/sundeep110/groundingLLMs
Happy Grounding!!
\ \ \
What's Your Reaction?
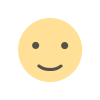
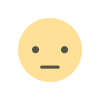
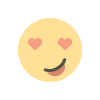
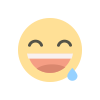
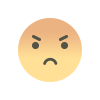
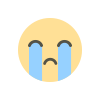
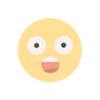