New Study from JNU Researchers Shows ClassBD Outperforms Other Fault Diagnosis Methods
This study compares multiple blind deconvolution (BD) methods for fault diagnosis, showing that the ClassBD method outperforms others in extracting fault features and handling noise.

Table of Links
2. Preliminaries and 2.1. Blind deconvolution
2.2. Quadratic neural networks
3.1. Time domain quadratic convolutional filter
3.2. Superiority of cyclic features extraction by QCNN
3.3. Frequency domain linear filter with envelope spectrum objective function
3.4. Integral optimization with uncertainty-aware weighing scheme
4. Computational experiments
4.1. Experimental configurations
4.3. Case study 2: JNU dataset
4.4. Case study 3: HIT dataset
5. Computational experiments
5.2. Classification results on various noise conditions
5.3. Employing ClassBD to deep learning classifiers
5.4. Employing ClassBD to machine learning classifiers
5.5. Feature extraction ability of quadratic and conventional networks
5.6. Comparison of ClassBD filters
5. Computational experiments
5.1. Comparison of BD methods
Despite the recent proposal of many BD methods, a few works have validated their fault diagnosis performance via the integration of classifiers. Initially, we assess the performance of these BD methods by employing WDCNN as the post-BD classifier. For comparative analysis, we utilize four prior-free BD methods: minimum entropy deconvolution (MED) [26], sparse maximum harmonics-to-noise-ratio Deconvolution (SMHD) [82], improved maximum correlated kurtosis deconvolution (IMCKD) [83], and multi-task neural network blind deconvolution (MNNBD) [31]. The metric employed for this evaluation is also the F1 score.
\ The classification results of the three datasets are presented in Table 8. Collectively, our approach outperforms others on all three datasets, indicating that the classifier-guided optimization is more apt for fault diagnosis. This is because traditional BD methods employ unsupervised optimization, whereas our method implements supervised learning, which introduces class-aware information. Furthermore, our approach is a streamlined one-step pipeline. When compared to the combination of conventional BD and classifiers, our method demonstrates superior effectiveness under noise-affected conditions.
\
\ On the other hand, it is imperative to validate the denoising performance of BD methods in the frequency domain, thereby demonstrating that these methods indeed extract fault-related features and provide interpretability. Therefore,
\
\ we design a frequency domain metric called fault frequency index (FFI), which is defined as follows:
\
\ Subsequently, we utilize the JNU dataset to demonstrate the feature extraction performance. The SNR is set to -10dB, and we apply BD methods to all faulty signals, and then calculate the FFI of the signals post-BD. The results are illustrated in Table 9. From these results, we can make several observations. Firstly, ClassBD is highly effective in extracting fault-related features, as evidenced by the highest FFIs across all signals, surpassing even the raw clean signals. Secondly, on average, all BD methods are capable of enhancing the fault characteristic frequencies. The FFIs are consistently higher than the noisy signals, with MNNBD ranking second-best, achieving an average improvement of 41% compared to the noisy signals.
\ Finally, we present a comparison of the feature extraction performance on the B1000 signal using Fast-SC. As depicted in Figure 7, the bright lines in the spectral coherence suggest that the magnitudes of the cyclic frequencies ???? and corresponding frequency bands are elevated. And the EES displays the intensity of the extracted cyclic frequencies of the signal post-BD. Several observations can be made from this. Firstly, the noise significantly attenuates the characteristics of the signal. A comparison between Figure 7 (a) and (b) reveals that the intensity of all frequency bands is suppressed, with the lower frequency bands being more severely drown out by the noise. Secondly, all BD methods are capable of enhancing the cyclic frequency characteristics of the signal, indicating the effectiveness of BD in
\
\ extracting fault-related features from noisy signals. Specifically, MNNBD focuses on the fundamental cyclic frequency, resulting in the extraction of full frequency bands at the first cyclic frequency. While SHMD and MED enhance all cyclic frequency bands, the amplitude of the individual cyclic frequencies is lower. Lastly, ClassBD exhibits the best performance. It significantly enhances the cyclic frequency characteristics of the signal. More importantly, ClassBD can provide valuable interpretability in decision-making.
\
\
:::info Authors:
(1) Jing-Xiao Liao, Department of Industrial and Systems Engineering, The Hong Kong Polytechnic University, Hong Kong, Special Administrative Region of China and School of Instrumentation Science and Engineering, Harbin Institute of Technology, Harbin, China;
(2) Chao He, School of Mechanical, Electronic and Control Engineering, Beijing Jiaotong University, Beijing, China;
(3) Jipu Li, Department of Industrial and Systems Engineering, The Hong Kong Polytechnic University, Hong Kong, Special Administrative Region of China;
(4) Jinwei Sun, School of Instrumentation Science and Engineering, Harbin Institute of Technology, Harbin, China;
(5) Shiping Zhang (Corresponding author), School of Instrumentation Science and Engineering, Harbin Institute of Technology, Harbin, China;
(6) Xiaoge Zhang (Corresponding author), Department of Industrial and Systems Engineering, The Hong Kong Polytechnic University, Hong Kong, Special Administrative Region of China.
:::
\
:::info This paper is available on arxiv under CC by 4.0 Deed (Attribution 4.0 International) license.
:::
\
What's Your Reaction?
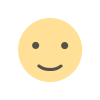
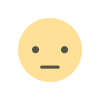
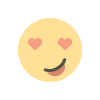
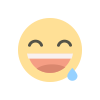
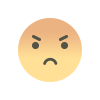
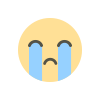
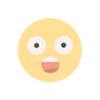